Manual Image Analyses
A portion of CERI revolves around detecting whether eelgrass beds have either recovered, deteriorated, or remained static over time. One way to conduct this research is to take samples via under water imagery. Researchers snorkel and kayak over eelgrass beds and take a bunch of photos which are then taken back to the lab. Once in the lab, the photos are analyzed by a technician to determine how much of the ocean floor is covered by eelgrass. As you can imagine, this task is extremely laborious so this is where Machine Learning comes into play!
CERI and Machine Learning
Machine learning pilot trials are underway to create a deep learning program that can classify percent cover of seagrass in photos. This program can be used to analyze the growth of seagrass (by % cover) over time and has application in restoration and long-term seagrass monitoring. If we are able to monitor the change in percent cover over time, we can assess whether the beds are growing back, or whether they are further depleting. Since manual analysis takes so much time for humans to do, an artificial intelligence program will make this process much more efficient!
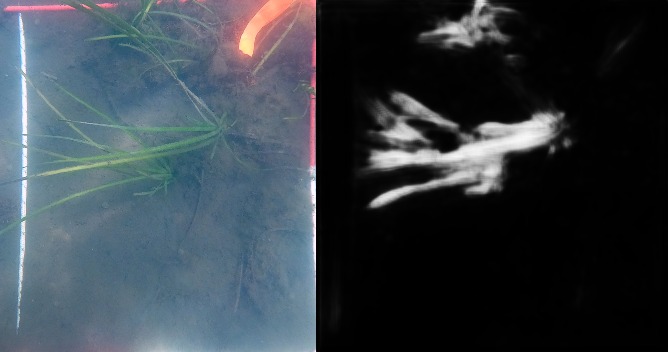
Image courtesy of the SEAM Lab at Dalhousie University.
Ben Misiuk from the Seascape Ecology and Mapping lab (SEAM) at Dalhousie has been working to create this A.I program that can help us classify percent eelgrass cover. Hannah Bordin, our previous Field Technician, worked with Ben on preliminary trials that tested the accuracy of this program. Using examples from humans, Ben is training an image recognition model (a neural network) to recognize eelgrass in imagery. Once the machine learns how to identify eelgrass, it can estimate what percentage of an image it covers. The survey can then be repeated and acquire new images to compare to the old ones.
Machine Learning set-up takes time, but once the model is trained with all of the imagery it will process quickly and efficiently.
This trial shows great promise for the future of A.I eelgrass restoration!